Younas Khan
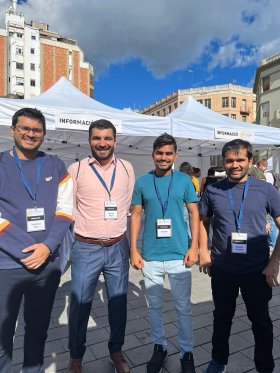
PhD Programme: Computer Science and Mathematics of Security
Research group: CRISES – Data security and privacy
Supervisors: Josep Domingo Ferrer & David Sánchez Ruenes
Bio
Mr. Younas Khan obtained his bachelor's degree in Computer Science from the Institute of Management Sciences Peshawar, in 2015, and his postgraduate degree from the National University of Sciences and Technology, Islamabad in 2019. During his PG he was able to work on numerous machine learning algorithms and was able to publish 5 international conferences, and develop two applications. After his PG, he taught in the University of Lahore, Lahore for a semester, and then in the Capital University of Science and Technology, Islamabad for two and a half years. During his 3 years of teaching experience, Mr. Khan taught numerous subjects including Procedural Programming, Object Oriented Programming, Data Structures, and Cryptography. Mr. Khan's current research interests include Machine Learning, Deep Learning, and Privacy.
Project: Synergies between machine learning and privacy
Modern societies produce and consume an enormous amount and variety of data on a daily basis. Even though the availability of such data is crucial in most areas of research, very often they contain personal information that cannot be shared with third parties or released publicly without adequate protection to ensure the fundamental right to privacy of the individuals they refer to. Classic data protection algorithms are only meant for structured data and/or require a significant amount of human intervention, which can hardly cope with the amount of data to be protected, and hence the need for fully automatic data protection is felt. On the other hand, modern machine learning has shifted from centralized architectures, in which a single trusted server compiles all the data and trains machine learning models, to decentralized settings, such as federated learning or fully decentralized learning, in which the learning effort is distributed among peers operating in a (typically open and untrusted) network. Even though these decentralized architectures alleviate the learning bottleneck at the server side, they are also more prone to suffer security and privacy attacks, due to the untrustworthiness of the participants and of the network itself. On the one hand, this implies leveraging state-of-the-art machine learning algorithms, such as transformers, embedding models and generative adversarial networks, to automate data protection and, in particular, the detection and masking of sensitive pieces of information in semi-structured or unstructured sources, or the generation of privacy-preserving synthetic data that faithfully represent the distribution of the original data. On the other hand, privacy-enhancing methods can be designed or adapted to decentralized machine learning scenarios, so that they are able to detect and filter out malicious peers, and to prevent or disable the privacy and security attacks those peers may orchestrate.